I’m of the same mind as people who think generative AI is grossly over-hyped by people who see it as their meal ticket. It is in many respects a big con, a solution desperately in search of problems, a product that was made and sold without a use case for it. Unfortunately, as many people have observed, the money being made off the hype is going to be used to destroy institutions, jobs and practices before the whole thing ebbs back to being whatever modestly or possibly useful thing it might turn out to be.
I hope that higher education withstands AI hype as much it (mostly) successfully held off the worst of the previous assaults by ed-tech speculators who insisted that massively-online courses were inevitably going to replace all brick-and-mortar education and usher in an era of cheap, plentiful, high-quality education available to everyone.
One thing that briefly produced a respite in the ed-tech assault was that for two years, we experienced a live demo of their best products and the results were bad for the most part. The technology was robust, the teachers actually did a pretty fair job with it, and here and there Zoom University worked out pretty well for students and faculty. There were small numbers of learners who were well-served by online instruction who don’t flourish face-to-face, and students who had mastered the habit of learning on their own powered through Zoom classes much as they would otherwise. There were pleasures in teaching from one’s own home as well, and new pedagogical tricks to try. So while the live demo showed that a fully online university is not the best for most people, not the right thing most of the time, there are things that are going to stick around that are enhancements of our work, not disruptive or destructive.
The problem is that the AI hypers in higher education are in many cases the same people who pushed all their chips in on ed-tech disruption. They’re desperate now to move their chips to the next hype while retaining the same destructive ambitions. They want to break the piggy bank, to get rid of higher ed as it is and own the Uber version of tomorrow. They’re pulling some of the same moves, too—creating shell organizations with public-facing leadership drawn from higher ed where all the funding and all the agenda is coming from a tech company desperate to make education be the use case that generative AI is searching for.
Right now the aspirant disruptors are selling (once again) the proposition that generative AI will replace expensive teachers or otherwise provision the fantasy of efficient frictionlessness that is so beloved of the proponents of austerity. Less publically, I think they’re also hoping that students and white-collar employees get addicted to using generative AI both licitly and illicitly in roughly the same way that the Sacklers were hoping that a lot of people with ankle sprains would get addicted to opoids. That’s what we have to get past. If we once again withstand the desire to demolish what works in favor of what won’t work, what might the enhancements be from the remnant of generative AI that sticks around afterwards?
I can imagine a future context in which generative AI is the equivalent of a herd animal that requires expert shepherds. Or where it’s a valuable—if specific and bounded—ecosystem that requires expert guides to navigate and work from. Or perhaps where it’s a kind of hazy alternative dimension that calls for wise shamans and gifted visionaries to act as intermediaries if you want to avoid dangerous spirits that lurk within.
What I’m reaching for in that cloud of metaphors is the idea of a future with generative AI where it’s a tool with limited but considerable uses in education, in culture-making, in processing information. Not the replacement for something as vast as “digital technology”, but maybe the enhancement of other intermediaries and tools. The word processor, for example, changed the process of writing in some very notable ways. Some writers at the dawn of word processing resented those transformations and contested them for a time. A few writers today continue to use alternative technologies to compose writing. But mostly we’ve accepted the word processor and embedded the changes in our collective understanding of the work of writing.
If I think about generative AI in those terms, one thing that occurs to me very strongly is that preparation right now for that possible and limited shift lies not in the adoption of some futuristic curriculum that is all computational but quite the opposite. It lies in much of what we already do, and most especially, in the humanities. And not just the part of the humanities that is about undertaking creative work but even more crucially the part that is about knowing about past cultural work.
In some sense this should be obvious about large-language-model AIs: they are trained on past writing or past artwork or existing code. This is also one of their weaknesses and I think will be a drawback for the foreseeable future. They are not really “machines that think”, they are reprocessors of what has been said and made. That’s where they have an unpleasant intersection with our present understanding of intellectual property and where they have a complicated intersection with our present cultivation of creativity and expression. It’s also where they embed much what it is our culture writ large even when we pretend otherwise—they have been trained on a vast corpus that includes racism, sexism, violence and many other sins and omissions. I understand the point that human beings are also reprocessors of what has been said and made, that this is sometimes what we mean by creativity—that someone has taken past work and thought of a new way to do it while still referencing what has come before. Really thinking in that process, however, remains ontologically, fundamentally different than what generative AI is doing.
If you want to really understand the outcomes of that corpus as reprocessed through generative AI, you can’t just be someone with a technical understanding of machine learning and large-language-models. It helps a great deal to understand culture, representation and interpretation, to know about the texts and textualities that have been used in the training.
Even more, it helps with the use of generative AI. If you look at something like Midjourney, the people who are consistently getting the most interesting and valuable results from it are people who combine several literacies at once: they know art history, they know about visual aesthetics, they know how generative AI generally works, and they know Midjourney as a tool and interface. They also often know where to find digital images and how to process and alter images in other tools. I’m not very good at any of things quite yet, but I understand how to push the tool closer to what I want as an image—from the generic outcomes of the generic prompt on the left side to more visually interesting and evocative images that might not be like anybody else’s first yield on the right side. There are people I see using it who are good at all of those things, and it shows.
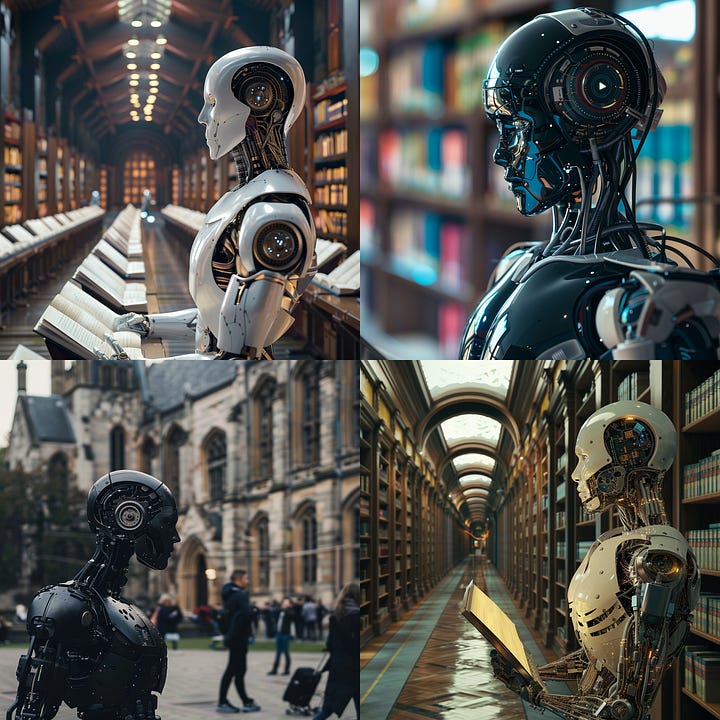
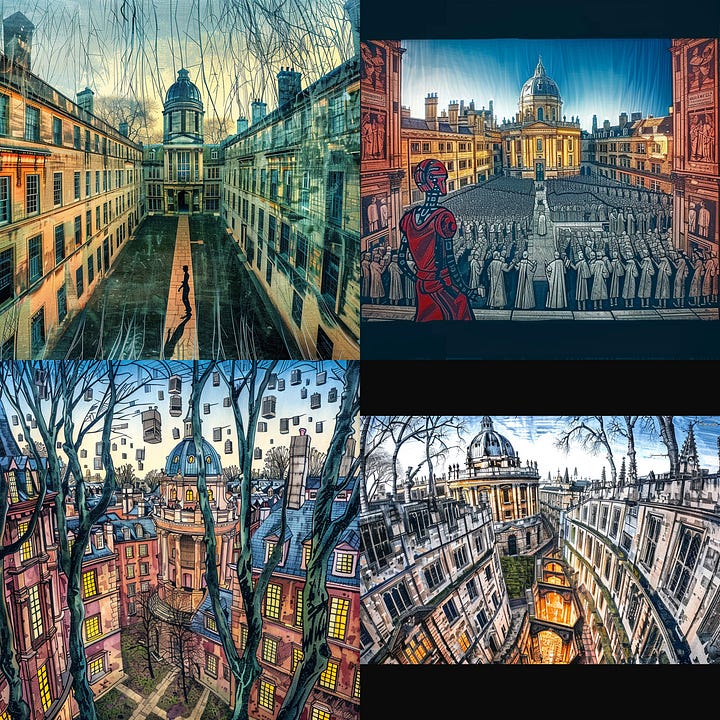
You are not going to be a great prompt engineer just from studying computer science. Nor will you be just by knowing art history. To go back to my metaphor, if we had to imagine a program of study for tomorrow’s users of the remnant useful leftovers after the AI hype has come and gone, if we are imagining training shepherds of AI, then that program of study will not be some exotic new thing. It will simply be a more effective connection between existing things than most universities and colleges are prepared to provide at this particular moment.
I've been re-reading One Useful Thing while writing a review of Co-Intelligence, Ethan Mollick's new book. Like you, and Alison Gopnick, Henry Farrell, and Brad Delong, Mollick points to how generative AI is at its core a cultural technology. "Magic for English Majors" is a good essay on this.
For all the supposed mystery of how LLMs are coming up with such good facsimiles of human thought or images, they are just just black boxes running statistical programs sitting on top of the internet. "Reprocessors of what has been said and made" is a good description. If you want value from using these reprocessors, as you say, you'd better know something about the history and social contexts of the steaming lumps of culture the machines regurgitate.
If only we could gather together people deeply knowledgeable about culture and history and have them offer educational experiences to young people interested in learning about culture and history, maybe we could...Nah! Let's spend trillions on building general intelligence, something Stephen Jay Gould long ago explained does not exist.